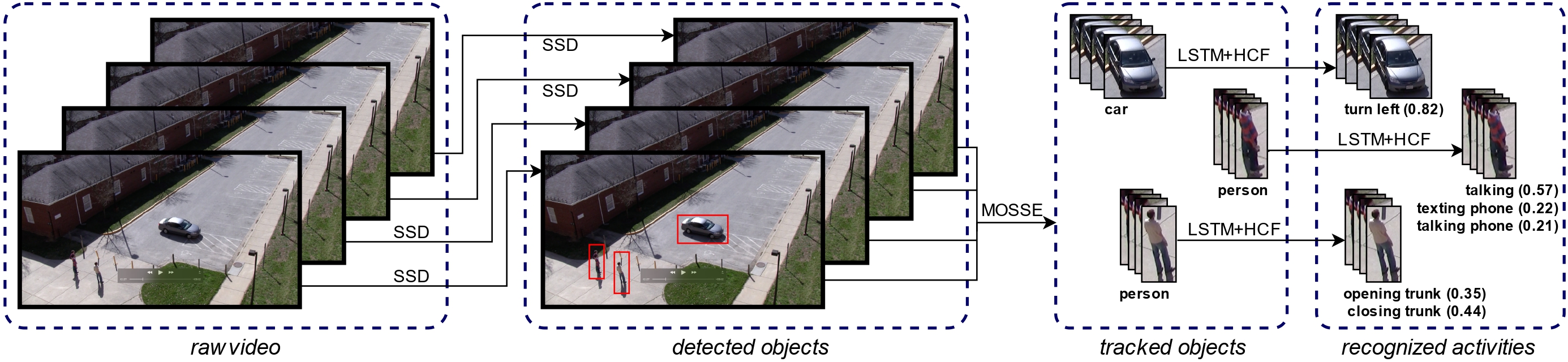
Event Detection in Surveillance Video
2018–2019
University of South Florida
2 Papers
A surveillance event is defined to be as one or more people performing a specified movement or interacting with an object or group of objects. We have developed a deep net framework for detection and tracking people and vehicles involved in various surveillance events in untrimmed extended video sequences.
TRECVID challenge participation
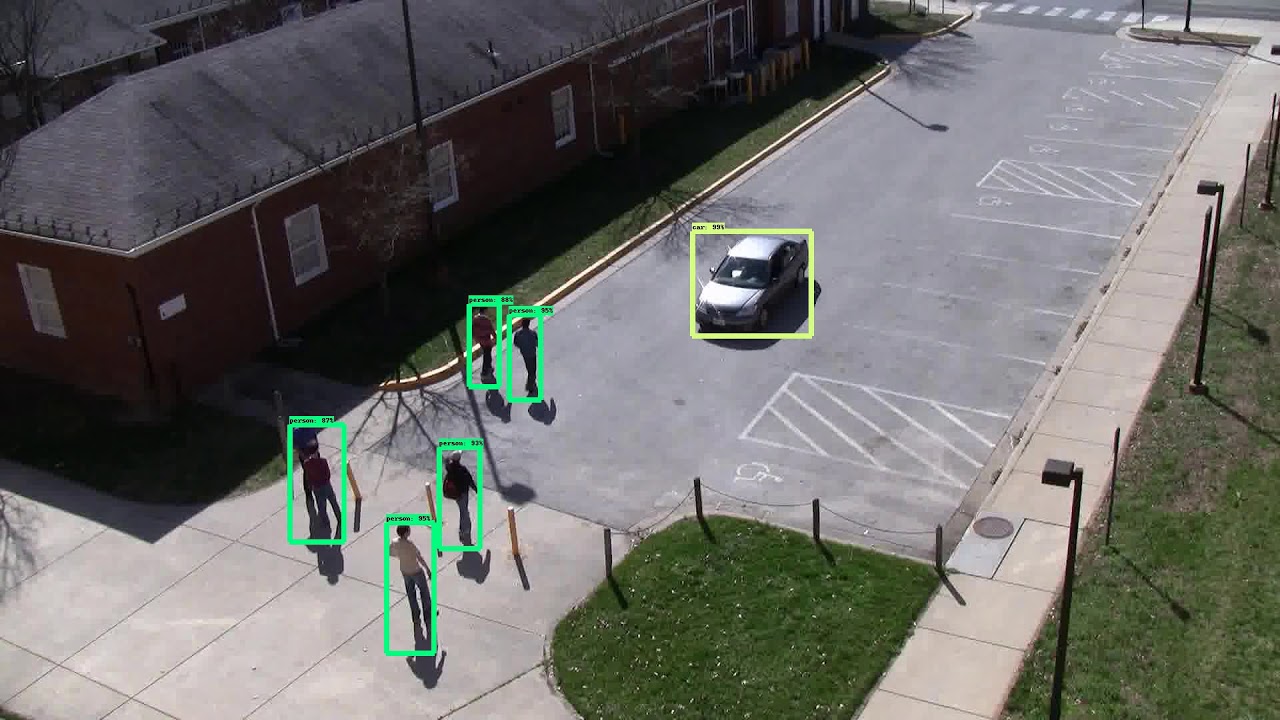
Our team USF Bulls participated in the 2018 TRECVID Activities in Extended Video Challenge with the goal to temporally and spatially localize a number of specific activities. A novel active object filter first discards large amounts of inactive data before the actual detection. Subsequently, the events are detected via proposal-based architecture with cascaded modules of a frame-wise object detector SSD, a probabilistic temporal tracker MOSSE and a recurrent neural net regressor LSTM with VGG16 features, followed by a set of hand-crafted filters. Addressing this problem was necessary to advance the development of robust, multi-camera, automatic activity detection algorithms for forensic and real-time alerting applications.
Spatio-temporal localization of activities in untrimmed surveillance videos presents a stiff challenge due to a large number of static and moving objects in the scene, objects involved in activities of arbitrary temporal duration and the fuzziness of interpretation of the activities. This method has introduced one original step in detection pipeline which filters out inactive objects, the so-called activeness regression. TRECVID winning models were based on the then-new 3DCNN model.
Evaluation on the VIRAT dataset shows that a proposed configuration yields 30% false alarms and 20% misdetections, which looks very promising and will improve with further research. At the TRECVID Challenge, our team was placed the 6th out of 27 teams. Gaining experience from this competition, the lab continued participating in this and similar challenges.
The project has a git repository. The work was published in two conference workshop proceedings.
© Michal Balazia. All Rights Reserved. Designed by HTML Codex