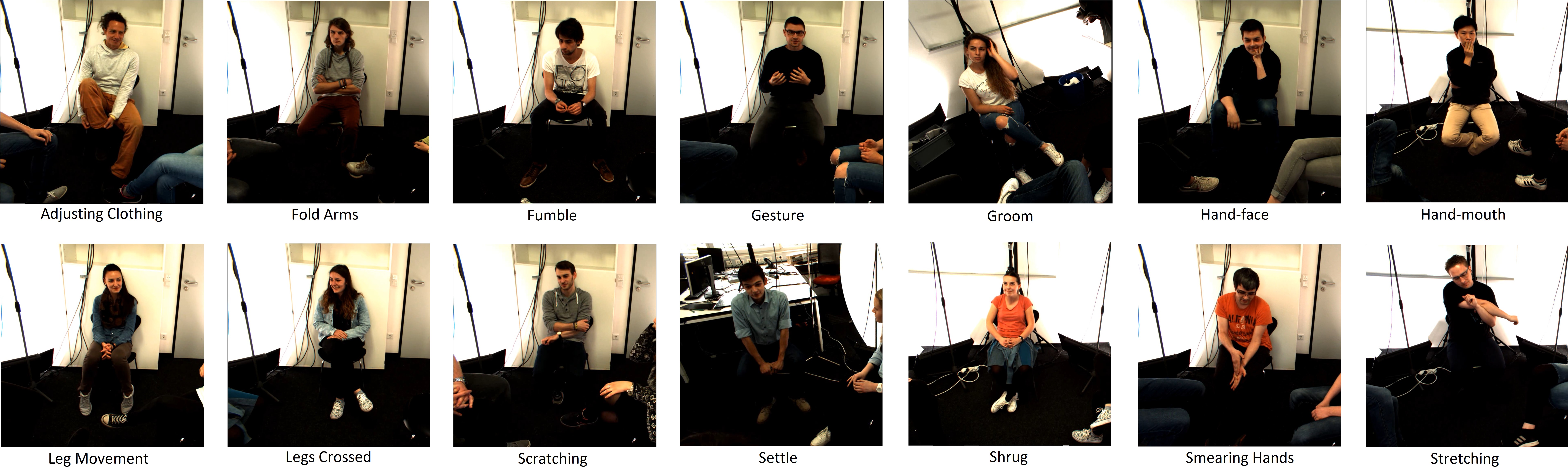
Body Behaviors in Social Interaction
2021–present
INRIA
3 Papers
Body language is an eye-catching social signal and its automatic analysis can significantly advance artificial intelligence systems to understand and actively participate in social interactions. While computer vision has made impressive progress in low-level tasks like head and body pose estimation, the detection of more subtle behaviors such as gesturing, grooming, or fumbling is not well explored.
Dataset MPIIGroupInteraction
We present the first set of annotations of complex Body Behaviors embedded in continuous Social Interactions (BBSI) in a group setting. Based on previous work in psychology, we manually annotated 26 hours of spontaneous human behavior in the MPIIGroupInteraction dataset with 15 distinct body language classes based on the the Ethological Coding System for Interviews (ECSI). This coding system includes many body behaviors that were shown to be connected to different social phenomena. We selected all ECSI behaviors involving the limbs and torso. In addition to the body behaviors included in ECSI, we scanned the MPIIGroupInteraction dataset for additional behaviors that occur frequently and carry potential meaning in a social situation. We present comprehensive descriptive statistics on the resulting dataset as well as results of annotation quality evaluations.
Detection of Body Behaviors
For automatic detection of the 15 behaviors, we adapt the Pyramid Dilated Attention Network (PDAN), a state-of-the-art approach for human action detection. We perform experiments using four variants of spatial-temporal features as input to PDAN: Two-Stream Inflated 3D CNN, Temporal Segment Networks, Temporal Shift Module and Swin Transformer. Results are promising and indicate a great room for improvement in this difficult task. Representing a key piece in the puzzle towards automatic understanding of social behavior, BBSI is fully available to the research community.
MultiMediate: Multimodal Behavior Analysis for Artificial Mediation
MultiMediate is a multi-year Grand Challenge at ACM Multimedia to contribute to realizing the vision of autonomous artificial mediators by measurable advances on key conversational behavior sensing and analysis tasks. The first iteration of MultiMediate in 2021 addressed eye contact detection and next speaker prediction while MultiMediate ’22 focused on backchannel analysis. MultiMediate ’23 introduced the tasks of body behavior recognition and engagement estimation. Engagement is a high-level phenomenon that is closely linked to previous years’ tasks, such as eye contact detection and backchanneling. The complex nature of engagement makes it prone to influences of context variables. Engagement might be expressed differently by people of different cultures, in different group compositions (dyadic vs. more than two people), and differently in professional vs. private contexts. In MultiMediate ’24 we pose the challenge of creating engagement estimation approaches that are able to transfer across such context factors.
To ensure continuing progress, we will also invite submission addressing the three most popular tasks of previous MultiMediate challenges: eye contact detection, backchannel detection, and body behavior recognition. Future iterations of the challenge will build on the achieved advances and focus on phenomena occurring at larger time scales, including escalation, liking, rapport, and dominance, and will make use of corpora currently being collected by the authors.
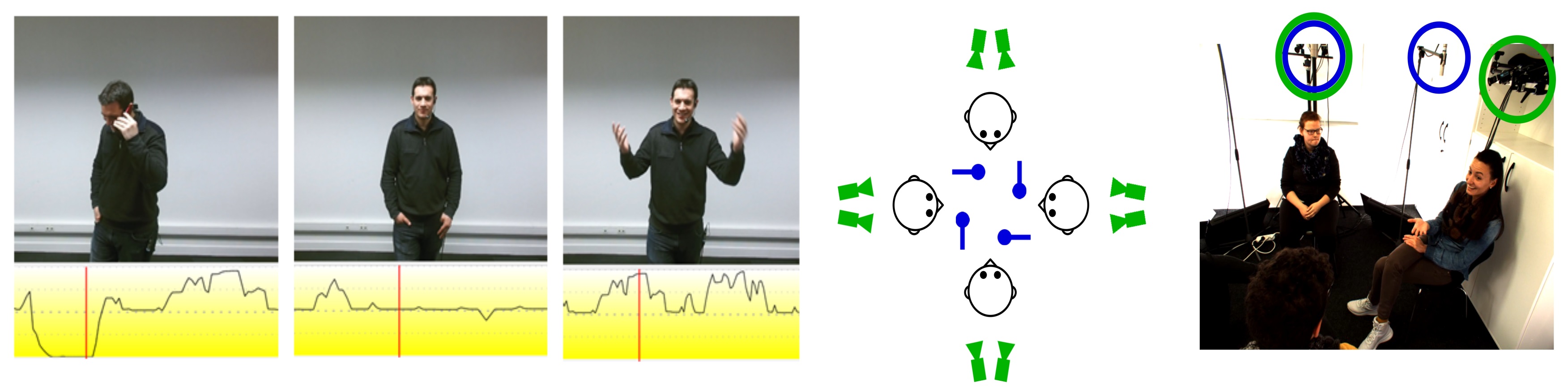
We have a strong collaboration with German Center for Artificial Intelligence, University of Stuttgart and Augsburg University. The work was published in six conference proceedings.
© Michal Balazia. All Rights Reserved. Designed by HTML Codex