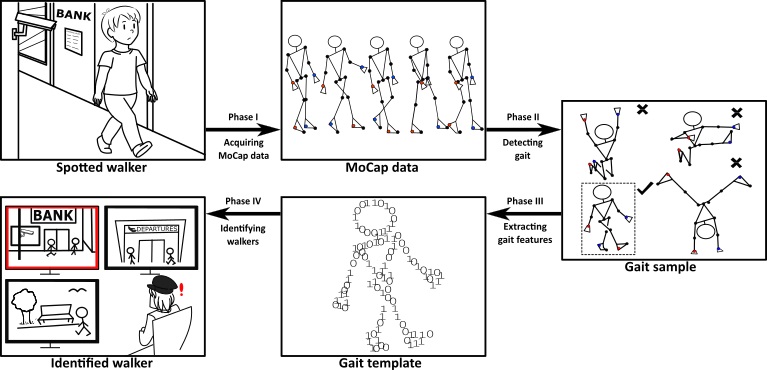
Gait Recognition from Motion Capture Data
2012–2022
Masaryk University
11 Papers
The goal of gait recognition is to identify individuals using walk pattern as a behavioral biometric. We have developed an end-to-end gait-assisted biometric recognition system to aid person identification and re-identification. The system includes new algorithms based on machine learning for detection of gait in general motion data, for extracting gait features, and for classification and clustering the identities.
Person identification and re-identification
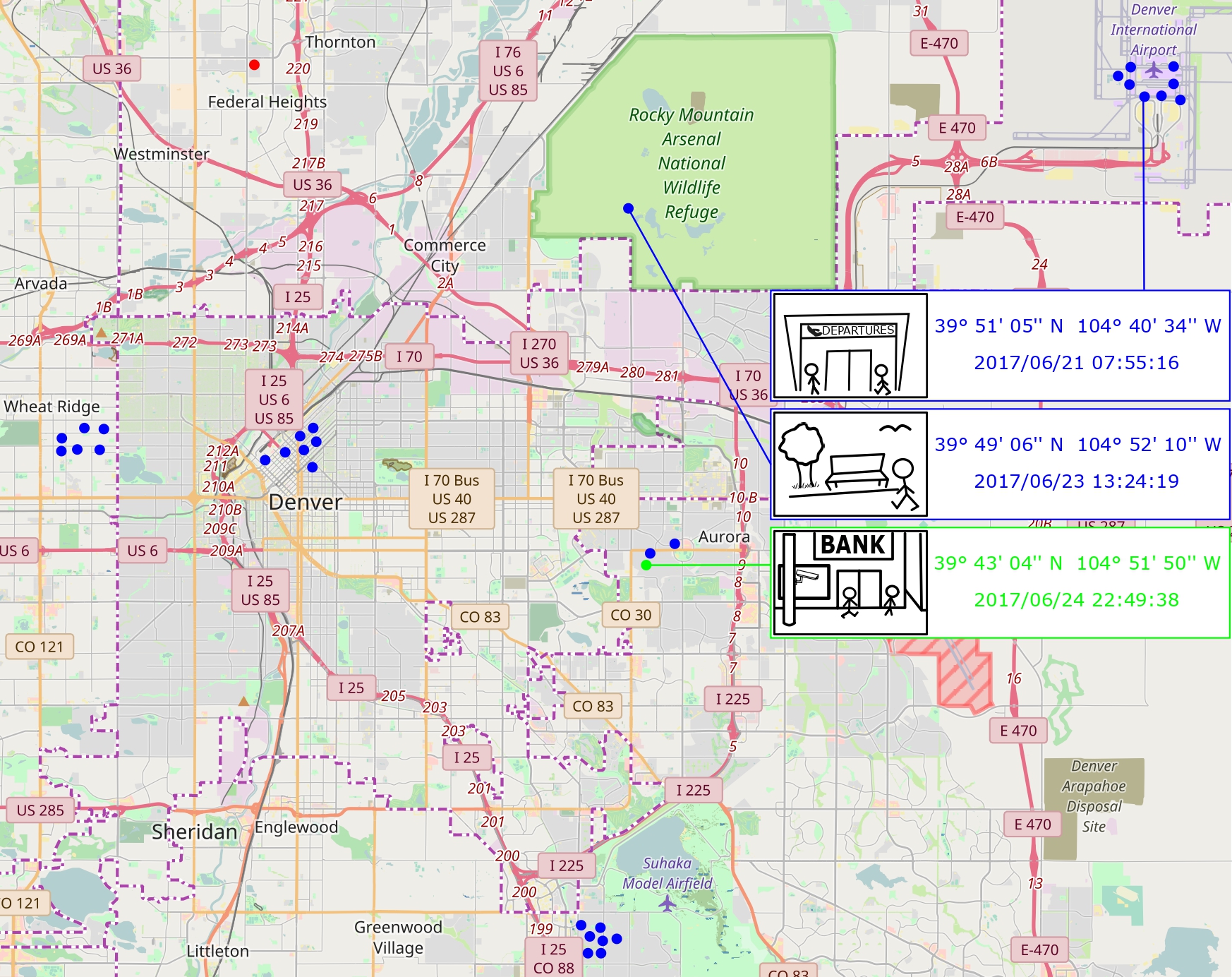
Latent features are trained by the Maximum Margin Criterion and by a combination of Principal Component Analysis and Linear Discriminant Analysis. Each of the introduced similarity models defines construction of a gait template from discriminative gait features, a measure for similarity of templates, and a decision mechanism for formulating recognition responses. In the identification context, the response is the label of the classified person's identity, while in the re-identification context, it is the cluster of gait samples collected at their incidents within the surveillance system. As a contribution to the reproducible research, my evaluation framework and database were made publicly available and used by other research teams.
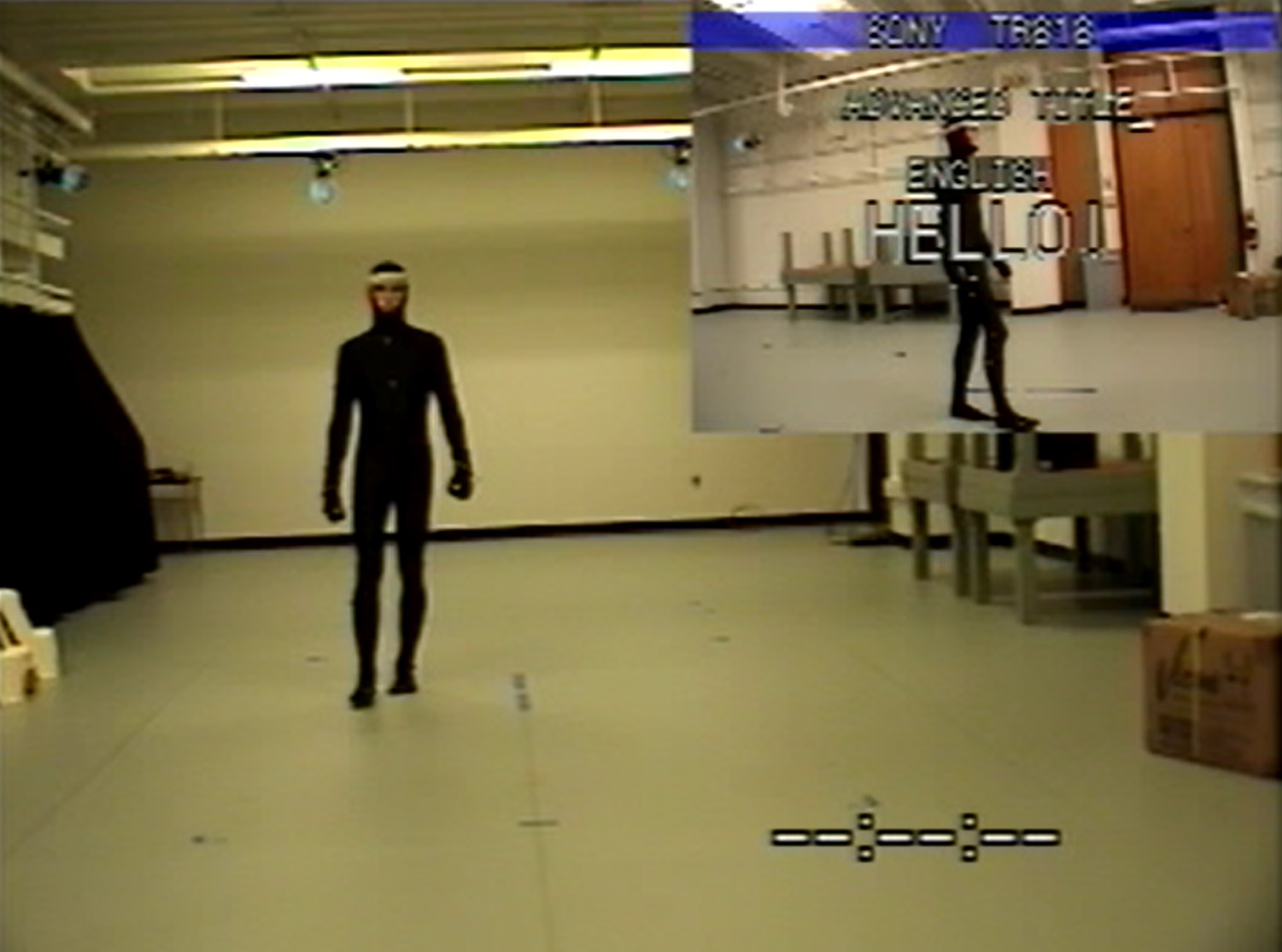
Introduced machine learning models advanced the state-of-the-art by a large step because the traditional approaches were based on hand-crafted classifiers of geometric features. These features often included joint angles, inter-joint distances, or the person's static body parameters such as height and bone lengths.
Evaluation on the CMU MoCap database of 5,923 samples and 64 identities shows that the latent features of the Maximum Margin Criterion method achieve 92.5% recognition rate. The framework has been used by about 8–10 other research teams.
The project has a web page and a git repository. It was published in many conference proceedings and journals and received multiple scientific awards: 2013 Swedish Innovation Prize, 2013 Diploma Thesis Award, 2018 IET Biometrics Premium Award, 2018 Joseph Fourier Prize, and 2019 Rector’s Award for an Outstanding Doctoral Thesis.
© Michal Balazia. All Rights Reserved. Designed by HTML Codex