|
Véronique Prinet
Ancien Chercheur invité, LIAMA, Beijing, China
Contact :
|
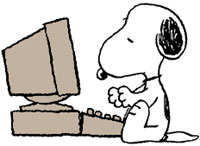
| Résumé :
S'il vous plait veuillez visiter ma page web ... |
Dernières publications dans le projet Ariana :
Extended Phase Field Higher-Order Active Contour Models for Networks. T. Peng et I. H. Jermyn et V. Prinet et J. Zerubia. International Journal of Computer Vision, 88(1): pages 111-128, mai 2010. Mots-clés : Contour actif, Champ de Phase, Shape prior, Parameter analysis, remote sensing, Road network extraction.
@ARTICLE{Peng09,
|
author |
= |
{Peng, T. and Jermyn, I. H. and Prinet, V. and Zerubia, J.}, |
title |
= |
{ Extended Phase Field Higher-Order Active Contour Models for Networks}, |
year |
= |
{2010}, |
month |
= |
{mai}, |
journal |
= |
{International Journal of Computer Vision}, |
volume |
= |
{88}, |
number |
= |
{1}, |
pages |
= |
{ 111-128}, |
url |
= |
{http://www.springerlink.com/content/d3641g2227316w58/}, |
keyword |
= |
{Contour actif, Champ de Phase, Shape prior, Parameter analysis, remote sensing, Road network extraction} |
} |
Abstract :
This paper addresses the segmentation from an image of entities that have the form of a ‘network’, i.e. the region in the image corresponding to the entity is composed of branches joining together at junctions, e.g. road or vascular networks. We present new phase field higher-order active contour (HOAC) prior models for network regions, and apply them to the segmentation of road networks from very high resolution satellite images. This is a hard problem for two reasons. First, the images are complex, with much ‘noise’ in the road region due to cars, road markings, etc., while the background is very varied, containing many features that are locally similar to roads. Second, network regions are complex to model, because they may have arbitrary topology. In particular, we address a limitation of a previous model in which network branch width was constrained to be similar to maximum network branch radius of curvature, thereby providing a poor model of networks with straight narrow branches or highly curved, wide branches. We solve this problem by introducing first an additional nonlinear nonlocal HOAC term, and then an additional linear nonlocal HOAC term to improve the computational speed. Both terms allow separate control of branch width and branch curvature, and furnish better prolongation for the same width, but the linear term has several advantages: it is more efficient, and it is able to model multiple widths simultaneously. To cope with the difficulty of parameter selection for these models, we perform a stability analysis of a long bar with a given width, and hence show how to choose the parameters of the energy functions. After adding a likelihood energy, we use both models to extract the road network quasi-automatically from pieces of a QuickBird image, and compare the results to other models in the literature. The state-of-the-art results obtained demonstrate the superiority of our new models, the importance of strong prior knowledge in general, and of the new terms in particular. |
Conditional mixed-state model for structural change analysis from very high resolution optical images. B. Belmudez et V. Prinet et J.F. Yao et P. Bouthemy et X. Descombes. Dans Proc. IEEE International Geoscience and Remote Sensing Symposium (IGARSS), Cape Town, South Africa, juillet 2009. Mots-clés : Change detection, mixed Markov models.
@INPROCEEDINGS{bel09,
|
author |
= |
{Belmudez, B. and Prinet, V. and Yao, J.F. and Bouthemy, P. and Descombes, X.}, |
title |
= |
{Conditional mixed-state model for structural change analysis from very high resolution optical images}, |
year |
= |
{2009}, |
month |
= |
{juillet}, |
booktitle |
= |
{IGARSS}, |
address |
= |
{Cape Town, South Africa}, |
url |
= |
{http://hal.archives-ouvertes.fr/inria-00398062/}, |
keyword |
= |
{Change detection, mixed Markov models} |
} |
Abstract :
The present work concerns the analysis of dynamic scenes from earth observation images. We are interested in building a map which, on one hand locates places of change, on the other hand, reconstructs a unique visual information of the non-change areas. We show in this paper that such a problem can naturally be takled with conditional mixed-state random field modeling (mixed-state CRF), where the ”mixed state” refers to the symbolic or continous nature of the unknown variable. The maximum a posteriori (MAP) estimation of the CRF is, through the Hammersley-Clifford theorem, turned into an energy minimisation problem. We tested the model on several Quickbird images and illustrate the quality of the results. |
An extended phase field higher-order active contour model for networks and its application to road network extraction from VHR satellite images. T. Peng et I. H. Jermyn et V. Prinet et J. Zerubia. Dans Proc. European Conference on Computer Vision (ECCV), Marseille, France, octobre 2008. Mots-clés : Dense urban area, Champ de Phase, Reseaux routiers, Methodes variationnelles, Very high resolution. Copyright :
@INPROCEEDINGS{Peng08c,
|
author |
= |
{Peng, T. and Jermyn, I. H. and Prinet, V. and Zerubia, J.}, |
title |
= |
{An extended phase field higher-order active contour model for networks and its application to road network extraction from VHR satellite images}, |
year |
= |
{2008}, |
month |
= |
{octobre}, |
booktitle |
= |
{Proc. European Conference on Computer Vision (ECCV)}, |
address |
= |
{Marseille, France}, |
pdf |
= |
{http://kepler.ia.ac.cn/publications/pub/2008/Peng-ECCV08.pdf}, |
keyword |
= |
{Dense urban area, Champ de Phase, Reseaux routiers, Methodes variationnelles, Very high resolution} |
} |
Abstract :
This paper addresses the segmentation from an image of entities that have the form of a 'network', i.e. the region in the image corresponding to the entity is composed of branches joining together at junctions, e.g. road or vascular networks. We present a new phase field higher-order active contour (HOAC) prior model for network regions, and apply it to the segmentation of road networks from very high resolution satellite images. This is a hard problem for two reasons. First, the images are complex, with much 'noise' in the road region due to cars, road markings, etc., while the background is very varied, containing many features that are locally similar to roads. Second, network regions are complex to model, because they may have arbitrary topology. In particular, we address a severe limitation of a previous model in which network branch width was constrained to be similar to maximum network branch radius of curvature, thereby providing a poor model of networks with straight narrow branches or highly curved, wide branches. To solve this problem, we propose a new HOAC prior energy term, and reformulate it as a nonlocal phase field energy. We analyse the stability of the new model, and find that in addition to solving the above problem by separating the interactions between points on the same and opposite sides of a network branch, the new model permits the modelling of two widths
simultaneously. The analysis also fixes some of the model parameters in terms of network width(s). After adding a likelihood energy, we use the model to extract the road network quasi-automatically from pieces of a QuickBird image, and compare the results to other models in the literature. The results demonstrate the superiority of the new model, the importance of strong prior knowledge in general, and of the new term in particular. |
|
Liste complète des publications dans le projet Ariana
|
|