Photonics design assisted by Deep Neural Networks
Harnessing the power of artificial intelligence to design next-generation photonic devices
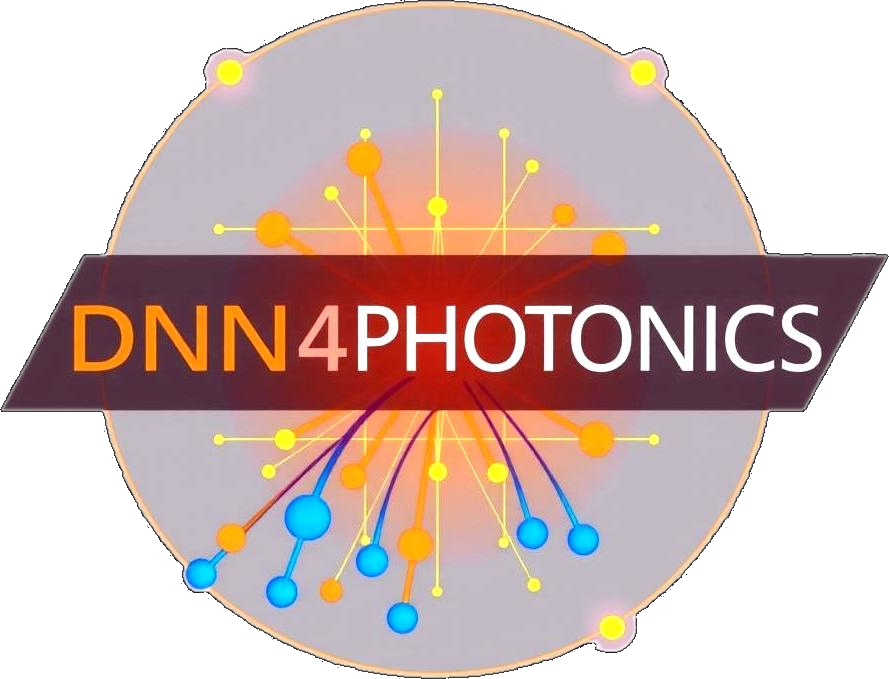
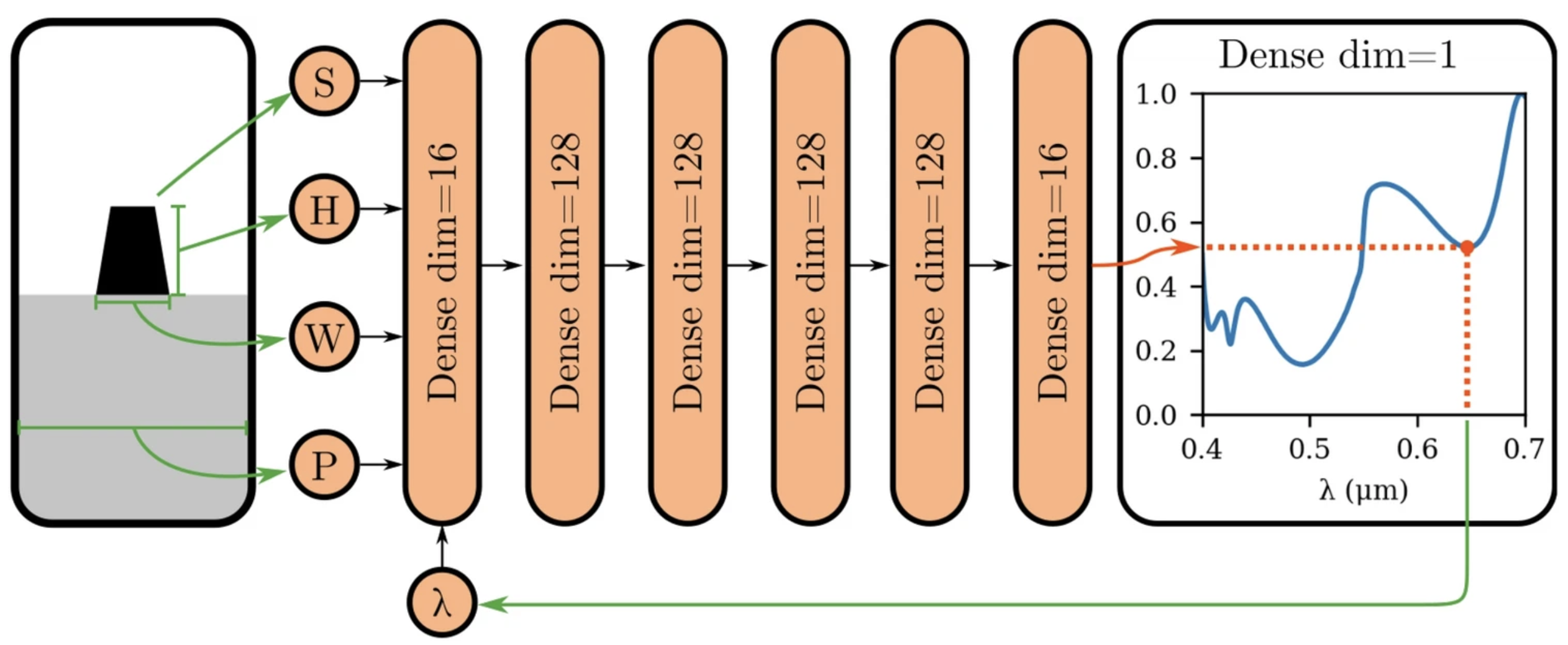
DNN4Photonics objectives
- Innovative methodologies using Deep Neural Networks (DNNs) for modeling and optimizing large-scale photonic structures
- Rapid and reliable surrogates for complex simulations, and efficient inverse design strategies
- Applications to the design of large-scale metasurfaces and metalenses
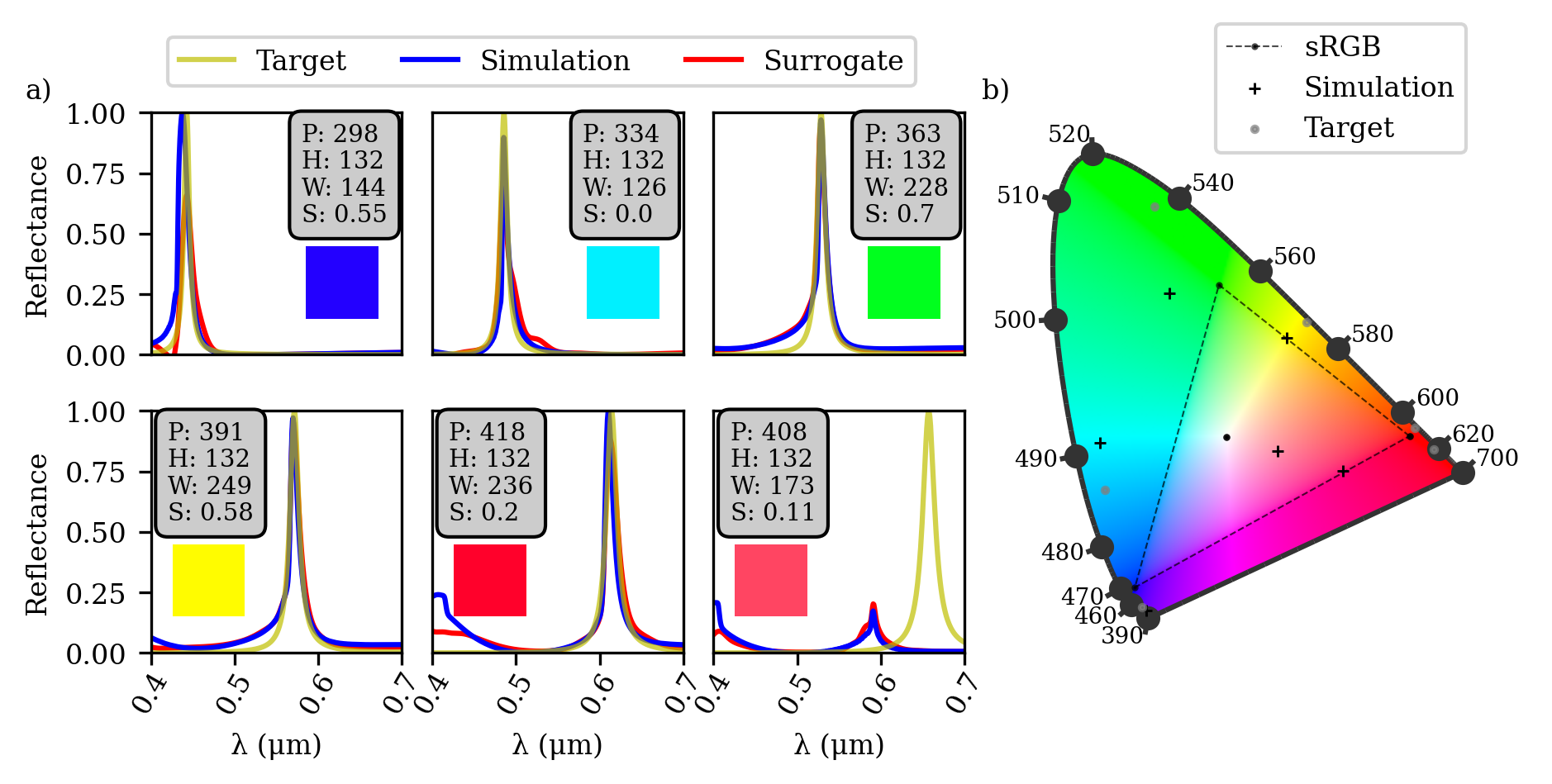
DNN4Photonics corresponds to two complementary projects: an Inria Associate Team that was initiated in January 2024, and a collaborative research project funded by ANR and FAPESP that has started in January 2025.
Overall, we aim at studying and developing disruptive approaches based on Deep Neural Networks for modeling and shaping the interactions of optical waves with matter when the latter is structured at the subwavelength scale. Electronic devices that exploit these interactions in their design are called photonic devices and the science that studies nanoscale light-matter interactions is referred as nanophotonics. Instead of solely resorting to classical numerical methods such as the finite element method for simulating these interactions, we propose to investigate data-driven Deep Learning methodologies for two main objectives: (1) devising fast and reliable surrogates of light wave propagation in the general case of three-dimensional spatial domains with complex scattering objects and, (2) devising inverse design strategies of photonic devices with unprecedented properties.
Meetings
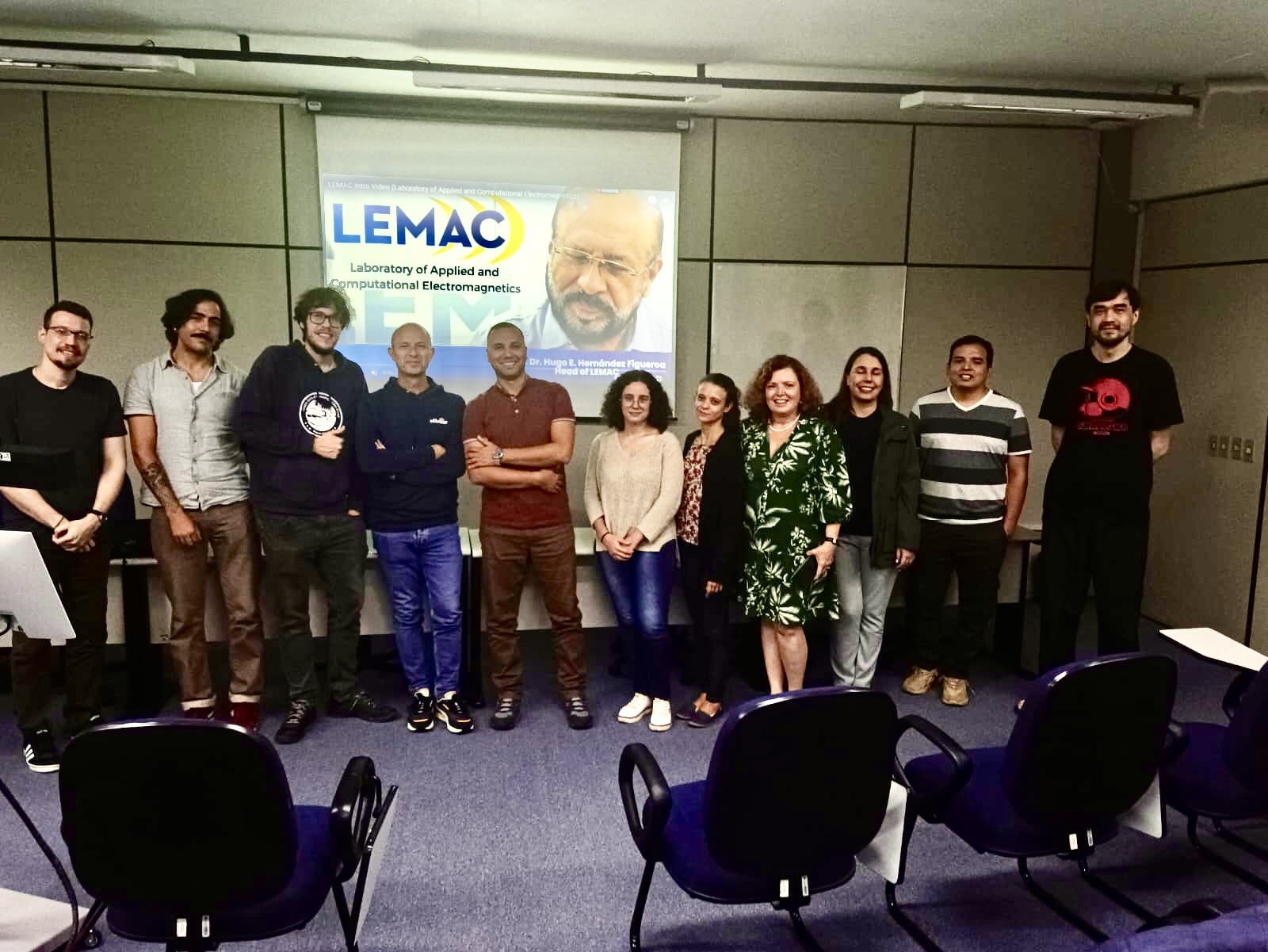
November 2024
First meeting of the DNN4Photonics Associate Team
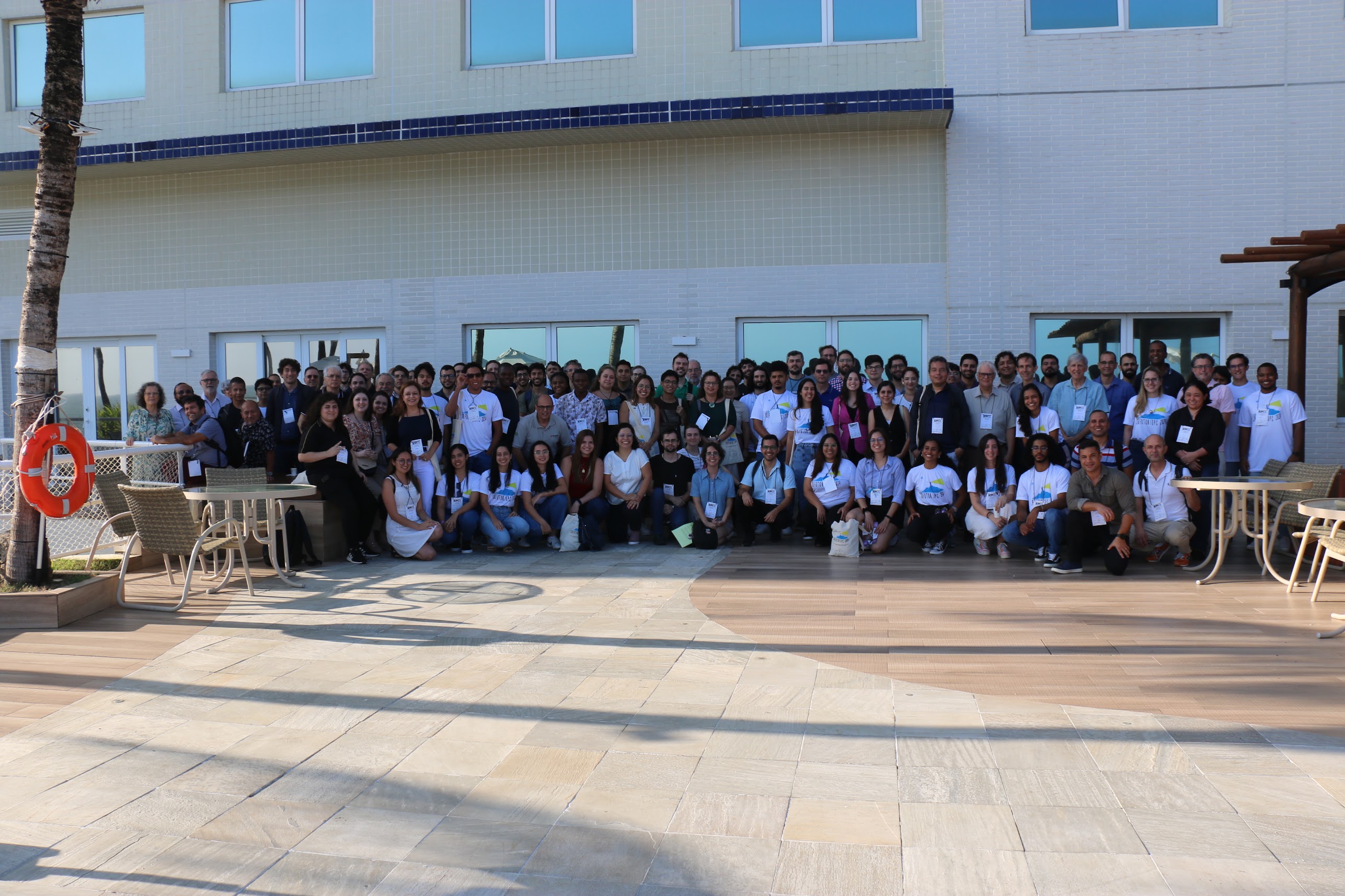
November 2024
Participation to SBFoton
2024 SBFoton International Optics and Photonics Conference
Salvador, State of Bahia, Brazil, from November 11th to 13th, 2024
Achievements
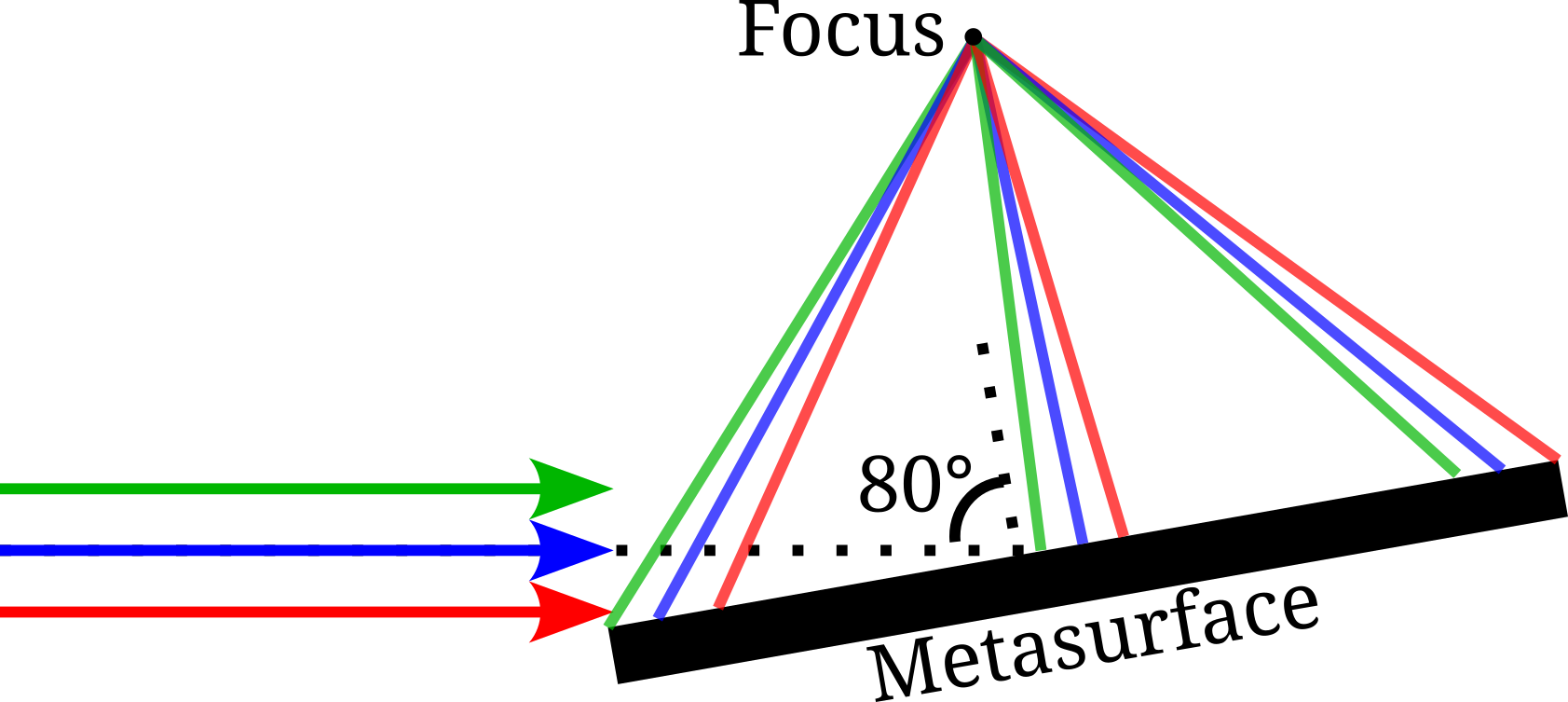
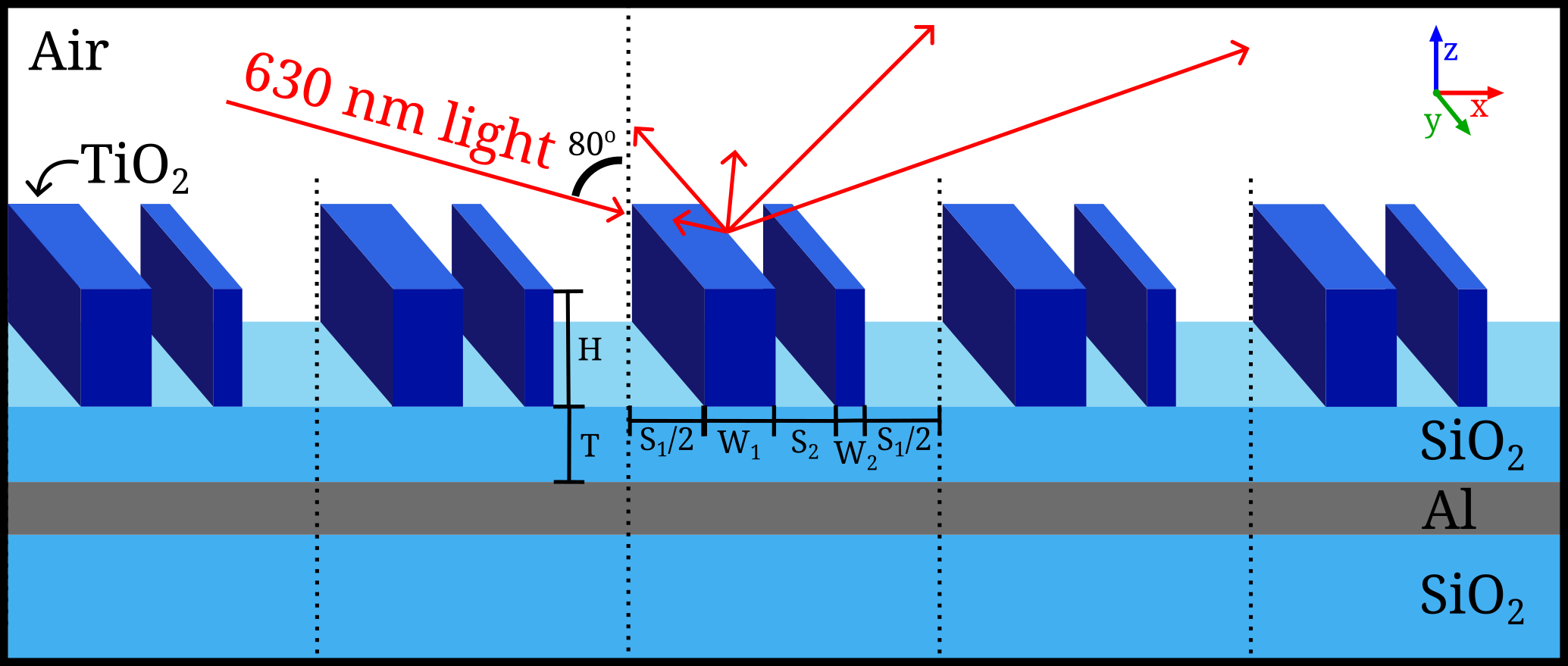
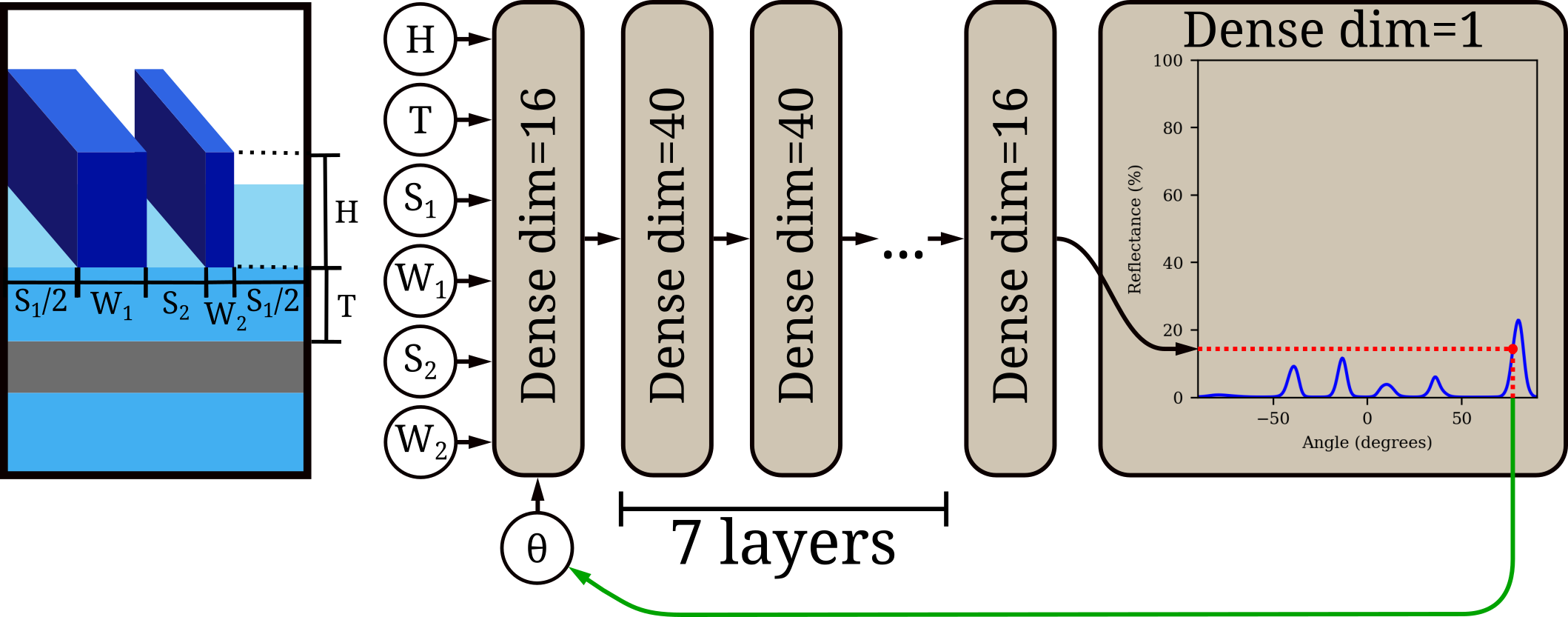
October 2024
Inverse design of metasurface-based beam deflectors
We develop an efficient inverse design methodology to conceive metasurface-based beam deflectors. We start by creating a small dataset composed by the results of simple simulations to train two deep neural networks. Once the models are trained, various beam deflectors can be inverse designed without further fullwave simulations. Our approach combines physical intuition about blazed gratings with a state-of-the-art optimization methodology, achieving high efficiencies while requiring modest computational effort. One design was successfully fabricated using sub-20 nm thermal scanning probe lithography.

Developed by the Atlantis project-team from Inria center at Université Cote d'Azur
Links
- Home
- About
- Team Hiring!
- All rights reserved.