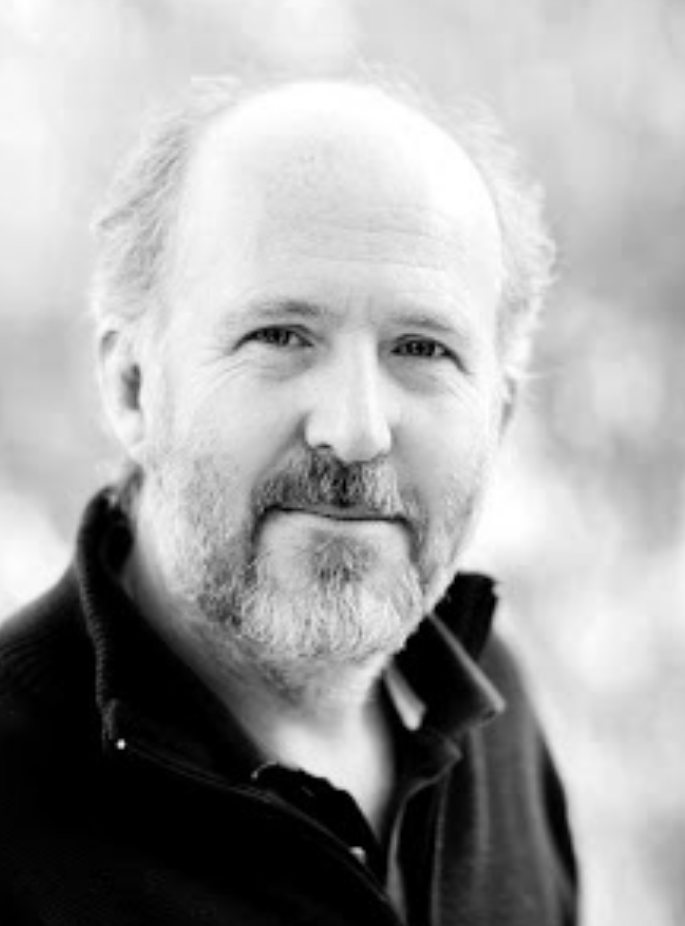
|
|
Xavier Pennec
|
Riemannian geometric statistics
In medical imaging in particular, but also in many other domains, the data to be processed only exceptionally have a linear structure. For instance, the core methods of computational anatomy rely on the statistical analysis of shapes, that are equivalence classes of sets of points, curves, surfaces or functions (images) by the action of a group of transformations (general positioning or reparameterization) and their structure is non-linear. Deformations, on the other hand, live in Lie groups. These objects have an intrinsic variability that we want to study and are perturbed by measurement noise. The statistical analysis of shapes and their deformations therefore requires a consistent statistical framework on manifolds, Lie groups and more general geometric structures: this is the objective of geometric statistics. The geometric structure that mainly considered are more specially Riemannian manifolds and Lie groups, but one can also consider stratified geodesic spaces.
The first statistical tool is the notion of central value of a distribution. In the 1930s-40s, Maurice Fréchet was one of the very first to attempt to compute the average of random curves. He observed that the expectation is a linear operator, and therefore cannot take value in a non-linear manifold. This leads him to redefine the mean in a metric space as the minimization of an intrinsic quantity: the expectation of the square of the distance to the data points. This definition was studied on Riemannian manifolds by H. Karcher then W. Kendall in the 1970s/80s, in particular for the characterization of uniqueness, and developed by D.G Kendall & K. Mardia in the community of directional statistics and shape statistics.
Simple statistics on Riemannian manifolds
In the field of signal and image processing, I proposed in my [PhD, 1996, (in French)] the use of the Fréchet mean and the associated estimation tools (least squares estimation, Kalman filter), with in particular the case of rotations and rigid transformations in 3D.
This was later reformulated as an intrinsic statistical theory on manifolds in the papers below. By considering the image of the distribution by the inverse of the exponential in the space tangent to the Fréchet mean, one can define the covariance matrix and the higher order moments. The Mahalanobis distance (distance weighted by the inverse of the covariance matrix) retains very general properties since its expectation is always equal to the dimension of the variety. Finally, I proposed to define the Gaussian on manifolds as the distribution maximizing the intrinsic Riemannian entropy knowing the mean and the covariance. In simple cases, it is a normal density with respect to the Riemannian measure in space tangent at the mean, truncated at the cut-locus. The concentration matrix parameterizing the dispersion in the exponential is still linked to the inverse of the covariance matrix, as in Euclidean spaces, but with a correction term involving the Ricci curvature. This entropic definition seems original and differs from the wrapped Gaussian considered in directional statistics and from the heat kernel on manifolds, even if the three notions converge for very small dispersions.
- X. Pennec. Intrinsic Statistics on Riemannian Manifolds: Basic Tools for Geometric Measurements. Journal of Mathematical Imaging and Vision, 25(1):127-154, July 2006. [PDF]
Keywords: Fréchet mean, Covariance matrix, Mahalanobis distance, Entropic characterization of Gaussians and Chi-square on manifolds.
[850 citations].
A preliminary (short) version was published at the NSIP 1999 conference[around 200 citations].
- X. Pennec, N. Ayache Uniform distribution, distance and expectation problems for geometric features processing. Journal of Mathematical Imaging and Vision 9 (1), 49-67, 1998. [PDF]
Keywords: Haar measure, invariant distance, Fréchet mean.
PCA on manifolds using flags of non-local barycentric subspaces
In order to model more than just the central value, principal component analysis (PCA) in the tangent space (tangent PCA) is the natural tool with the above statistical framework. However, if this is often sufficient for analysing data which are sufficiently centred around a central value (unimodal or Gaussian-like data), it fails for multimodal or large support distributions. Generalizations like Principal Geodesic Analysis (PGA) or geodesic PCA minimizing the distance to geodesic subspaces have been proposed to handle more variability. However, since geodesic subspaces are generated by geodesics tangent to a subspace of the tangent space at a given point (the mean for PGA, a point on the principle geodesic for GPCA), these methods remain quite local and very sensitive to that development point. Other methods such as Principal Nested Spheres (PNS) highlight the importance of nesting the principal subspaces generated, but remain limited to very simple manifolds.
In this work, I first propose a new non-local family of subspaces in manifolds, call barycentric subspaces. They are implicitly defined as the locus of points which are the weighted average of reference points (generalization of the affine space generated by points). As this definition relies on points and not on tangent vectors, it can also be extended to geodesic spaces which are not Riemannian. For instance, in stratified metric spaces, it naturally allows to have principal subspaces that span over several strata, which is not the case with PGA. Barycentric subspaces locally define a submanifold of dimension k which generalizes the geodesic subspaces used in the above methods. Like Euclidean subspaces in PCA or geodesic subspaces in PGA, barycentric subspaces can naturally be nested. Thus, ordering the points produces a natural filtration of nested barycentric subspaces, that is: a flag of non-linear subspaces (a hierarchies of properly embedded subspaces of increasing dimension).
PCA is most often viewed as an iterative procedure constructing inductively this flag of nested subspaces starting from the mean and approximating data points gradually better at each step. This forward procedure is in use for PGA and GPCA, while PNS is based on a backward that may not converge to the mean. In the non-linear cases, these forward or backward procedures are not consistent with the optimal subspace at each dimension. The second major contribution of this work is to show that classical PCA is actually optimizing a criterion on the space of linear flags: the accumulated unexplained variance. Seeing PCA as an optimization and no longer as an iterative method opens up many perspectives for machine learning. This criterion also generalizes very naturally to flags of barycentric subspaces in Riemannian manifolds. This results into a particularly appealing generalization of PCA on manifolds, that is called Barycentric Subspaces Analysis (BSA).
With MM Rohé, this framework was adapted to image registration to build low-dimensional representation of the cardiac motion from MRI image image sequences. We could demonstrate that choosing 3 images as reference point along the sequence was not only sufficient to reconstruct the sequence very precisely, but also that the barycentric coordinates defined a meaningful signature for group-wise analysis of dynamics that can efficiently separate two populations.
- MM Rohé, M Sermesant, X Pennec.
Low-dimensional representation of cardiac motion using barycentric subspaces: a new group-wise paradigm for estimation, analysis, and reconstruction. Medical image analysis 45, 1-12, 2018.
DOI : 10.1016/j.media.2017.12.008.
[PDF]
Effet of curvature on the concentration of the empirical mean in manifolds
The Bhattacharya and Patrangenaru central limit theorem (BP-CLT) establishes the concentration of the Fréchet mean of IID random variables on a Riemannian manifold with a high number of samples. This asymptotic result shows that the Fréchet mean behaves almost as the usual Euclidean case for sufficiently concentrated distributions. However, the asymptotic covariance matrix of the empirical mean is modulated by the inverse of the expected Hessian of the squared distance. This Hessian matrix was explicitly computed in a further work for constant curvature spaces in order to relate it to the sectional curvature. Although explicit, the formula remains quite difficult to interpret, and the intuitive effect of the curvature on the asymptotic convergence remains unclear. Moreover, we are most often interested in the mean of a finite sample of small size in practice.
In this work, we aim at understanding the effect of the manifold curvature in this small sample regime. Moreover, we aim at deriving computable and interpretable approximations that can be extended from the empirical Fréchet mean in Riemannian manifolds to the empirical exponential barycenters in affine connection manifolds. For distributions that are highly concentrated around their mean, and for any finite number of samples, we establish explicit Taylor expansions on the first and second moment of the empirical mean thanks to a new Taylor expansion of the Riemannian log-map in affine connection spaces. This shows that the empirical mean has a bias in 1/n proportional to the gradient of the curvature tensor contracted twice with the covariance matrix, and a modulation of the convergence rate of the covariance matrix proportional to the covariance-curvature tensor. We show that our non-asymptotic high concentration expansion is consistent with the asymptotic expansion of the BP-CLT. Experiments on constant curvature spaces demonstrate that both expansions are very accurate in their domain of validity. Moreover, the modulation of the convergence rate of the empirical mean's covariance matrix is explicitly encoded using a scalar multiplicative factor that gives an intuitive vision of the impact of the curvature: the variance of the empirical mean decreases faster than in the Euclidean case in negatively curved space forms, with an infinite speed for an infinite negative curvature. This suggests potential links with the stickiness of the Fréchet mean described in stratified spaces. On the contrary, the variance of the empirical mean decreases more slowly than in the Euclidean case in positive curvature space forms, with divergence when we approach the limits of the Karcher and Kendall concentration conditions with a uniform distribution on the equator of the sphere, for which the Fréchet mean is not a single point any more.
Geometrization of statistics in path-metric spaces
In statistics, independent, identically distributed random samples do not carry a natural ordering, and their statistics are typically invariant with respect to permutations of their order. Thus, an n-sample in a space M can be considered as an element of the quotient space of Mn modulo the permutation group. The present paper takes this definition of sample space and the related concept of orbit types as a starting point for developing a geometric perspective on statistics. We aim at deriving a general mathematical setting for studying the behavior of empirical and population means in spaces ranging from smooth Riemannian manifolds to general stratified spaces. We fully describe the orbifold and path-metric structure of the sample space when M is a manifold or path-metric space, respectively. These results are non-trivial even when M is Euclidean. We show that the infinite sample space exists in a Gromov-Hausdorff type sense and coincides with the Wasserstein space of probability distributions on M. We exhibit Fréchet means and k-means as metric projections onto 1-skeleta or k-skeleta in Wasserstein space, and we define a new and more general notion of polymeans. This geometric characterization via metric projections applies equally to sample and population means, and we use it to establish asymptotic properties of polymeans such as consistency and asymptotic normality.
- Ph. Harms, P.W. Michor, X. Pennec, S. Sommer. Geometry of Sample Spaces.
Differential Geometry and its Applications, In press, 2023.
Xavier
Pennec