Computational Anatomy of the Brain
6. Example use of Computational Anatomic Models in the Clinical Workflow
6.1 Better Constrain Atlas-to-Patient Registration for Radiotherapy
The planning step for conformal radiotherapy requires the accurate localisation of the tumour, to maximise its irradiation, and of the critical structures where the irradiation has to be minimises. To segment these structures for each patient, one standard method is to register a previously labelled atlas to the patient image. This allows transferring the generic atlas segmentation toward the patient-specific space. This segmentation can then be used directly, or as an initialisation for a more complex segmentation algorithm [Commowick, 2007]. In such a system, the main difficulty is to obtain an inter-subject registration algorithm which is accurate enough and, more importantly, robust to the anatomical variability and to the pathologies (tumours may be quite important).
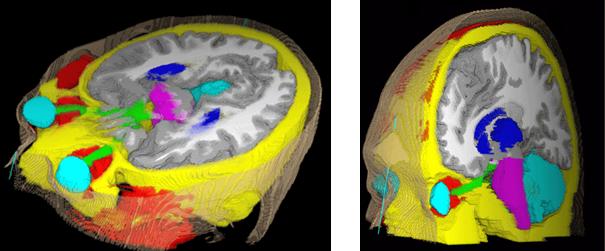
The main method to enforce meaningful deformations is to penalise non sensible ones through a regularisation criterion. Some authors used physical models like elasticity or fluid models [Bajcsy and Kovacic, 1989, Christensen et al., 1997]. For efficiency reasons, other authors proposed to use non-physical but fast regularisation methods like Gaussian filtering [Thirion, 1998, Pennec et al., 1999, Modersitzki, 2004]. However, since we do not have in general a model of the deformation of organs across subjects, no regularisation criterion is obviously more justified than the others. Thus, most of the existing work in the literature rather tries to capture the organ variability from a statistical point of view on a representative population of subjects (see e.g. [Thompson et al., 2000, Rueckert et al., 2003, Fillard et al., 2005]). For instance, following [Lester et al., 1999], the algorithm RUNA developed in [Stefanescu et al., 2004, Stefanescu, 2005] was a first attempt to obtain a computationally efficient but highly steerable nonlinear registration algorithm that includes some anatomical information about the tissue types. It uses a non-stationary transformation regularisation which is strong where the local deformability is expected to be low, and conversely. This also allows taking into account pathologies such as tumours or previous resections.
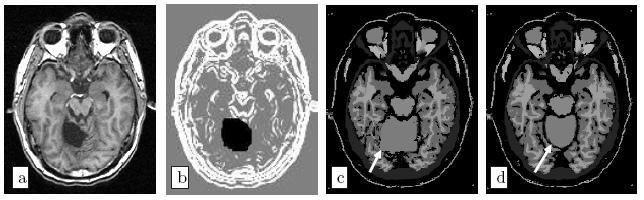
Moreover, the regularisation can be locally tuned along spatial directions through the use of a tensor field, as we did for instance in [Commowick et al., 2005], by introducing a method to compute scalar and tensor based deformability statistics over a database of patient.
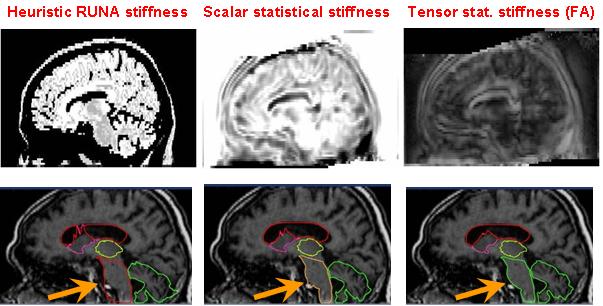
6.2 Mapping the fibres to patient images for tumour growth models
As shown in the tumour growth model section, the DTI information is crucial in the formulation of the tumour growth model because tumour cells move much faster on the white matter and they tend to follow the fibre tracts. Hence, the infiltration pattern of the tumour highly depends on the local fibre directions. Since diffusion tensor images give the average local fibre orientation they can be used to extract these tumour highways as it was done in the model we have explained to construct the diffusion tensor of tumour cells. Unfortunately, DT-MRI images are seldom acquired in the clinical routine because of technical constraints (old clinical MR scanners often do not have gradients powerful enough to acquire this sequence), time constraints which are crucial for the image quality, especially on children (a typical DTI acquisition lasts about 10 minutes during which the child should not move, which may require sedation), ethical issues and economical efficiency (there is no clinical indication for DTI yet).
Thus, in order to use local fibre directions information for patients without DTI, we have to rely on a generic atlas of fibres, i.e. register tensors from a healthy subject onto the patient anatomy.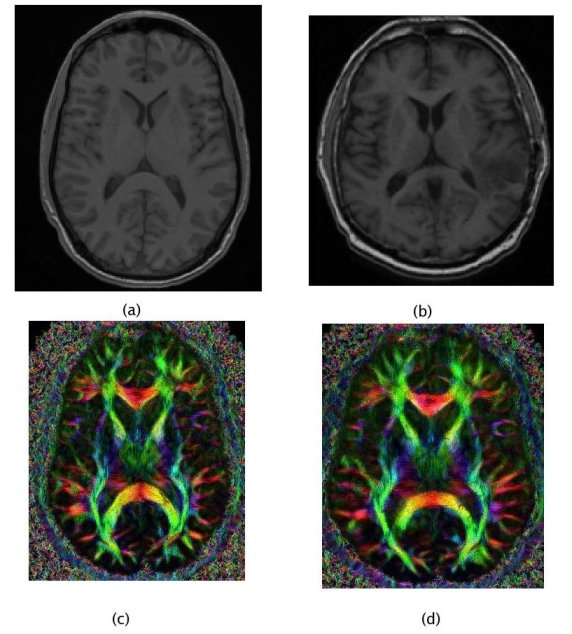
In such a personalisation process, the quality of the registration algorithm is essential as it directly affects the model and the growth simulation. Thus, using prior knowledge about the inter-subject variability is necessary to obtain a fibre mapping which is anatomically meaningful. Once again, computational anatomy appears as a key component of the clinical image analysis work-flow.
6.3 References
- [Bajcsy and Kovacic, 1989] Bajcsy R., Kovacic S., Multiresolution Elastic Matching, CVGIP, vol. 46, 1989, p . 1-21 .
- [Bondiau, 2004] Bondiau P.Y. Mise en oeuvre et évaluation d'outils de fusion d'image en radiothérapie. Thèse de sciences, Université de Nice-Sophia Antipolis, November 2004
- [Christensen et al., 1997] Christensen G., Joshi S., Miller M., Volumetric transformation of brain anatomy, IEEE Trans. Med. Imag., 16, 864--877, 1997
- [Commowick 2005] Commowick O., Stefanescu R., Fillard P., Arsigny V., Ayache N., Pennec X., and Malandain G. Incorporating Statistical Measures of Anatomical Variability in Atlas-to-Subject Registration for Conformal Brain Radiotherapy. In J. Duncan and G. Gerig, editors, Proceedings of MICCAI 2005, volume 3750 of LNCS, pages 927-934, 2005. Springer Verlag
- [Commowick, 2007] Commowick O., Design and Use of Anatomical Atlases for Radiotherapy. PhD Thesis, Nice -- Sophia-Antipolis University, February 2007
- [Fillard et al, 2007] Pierre Fillard, Vincent Arsigny, Xavier Pennec, Kiralee M. Hayashi, Paul M. Thompson, and Nicholas Ayache. Measuring Brain Variability by Extrapolating Sparse Tensor Fields Measured on Sulcal Lines. Neuroimage, 34(2):639-650, January 2007. Note: Also as INRIA Research Report 5887, April 2006. PMID: 17113311.
- [Lester, 1999] Lester, H., Arridge, S.R., Jansons, K.M., Lemieux, L., Hajnal J.V. and Oatridge, A. Non-linear Registration with the Variable Viscosity Fluid Algorithm. Proc of IPMI'99, LNCS 1613, p.238-251, 1999.
- [Modersitzki, 2004] Modersitzki J., Numerical Methods for Image Registration, Oxford University Press Series: Numerical Mathematics and Scientific Computation, 2004
- [Pennec et al, 1999] Xavier Pennec, Pascal Cachier, and Nicholas Ayache. Understanding the ``Demon's Algorithm'': 3D Non-Rigid registration by Gradient Descent. In C. Taylor and A. Colchester, editors, Proc. MICCAI'99, volume 1679 of LNCS, Cambridge, UK, pages 597-605, September 1999. Springer Verlag.
- [Rueckert et al, 2003] Rueckert D., Frangi A.F., Schnabel J.A., Automatic construction of 3-D statistical deformation models of the brain using nonrigid registration, IEEE Transactions on Medical Imaging 22,p. 1014- 1025, 2003
- [Stefanescu, 2004] Radu Stefanescu, Xavier Pennec, and Nicholas Ayache. Grid Powered Nonlinear Image Registration with Locally Adaptive Regularization. Medical Image Analysis, 8(3):325-342, September 2004. PMID: 15450226.
- [Stefanescu et al, 2005] Stefanescu R., Pennec X., and Ayache N. A Grid Service for the Interactive Use of a Parallel Non-Rigid Registration Algorithm of Medical Images. Methods of Information in Medicine, 44(2), 2005.
- [Thirion, 1998] Thirion J.P. Image matching as a diffusion process: An analogy with maxwell's demon. Medical Imag. Analysis, 2:243--260, 1998.
- [Thompson et al., 2000] Thompson, P., Mega, M., Narr, K., Sowell, E., Blanton, R., and Toga, A. (2000). Brain image analysis and atlas construction. Handbook of Medical Image Proc. and Analysis, chapter 17. SPIE.